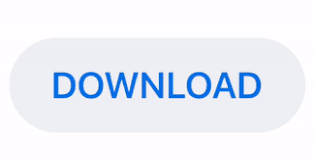
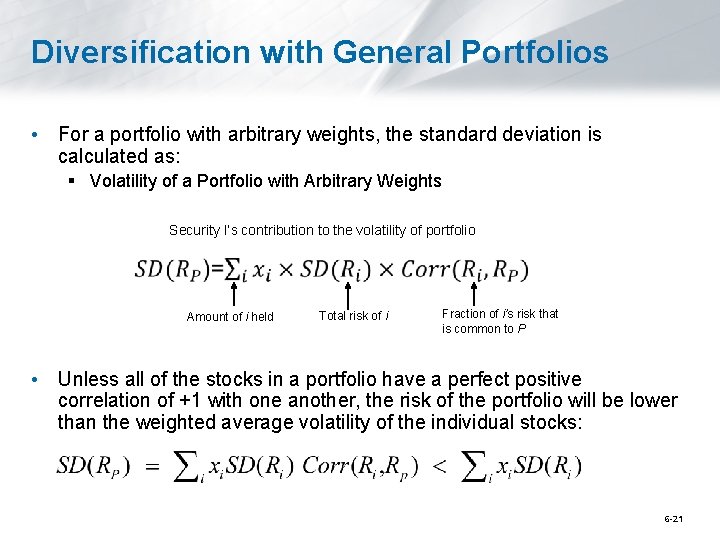
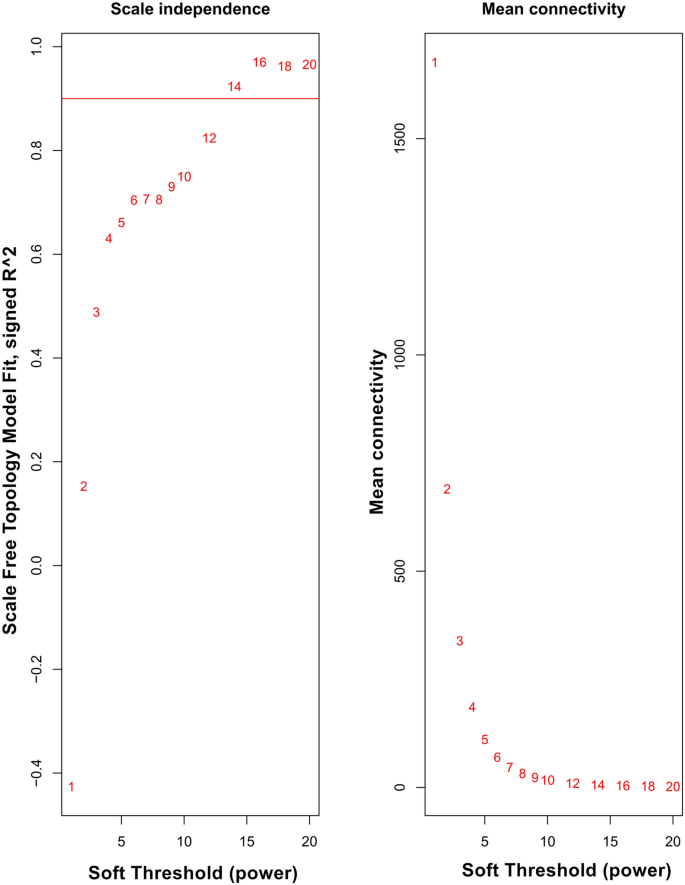
The latter has more features but also represents a more massive dependency in your code. There is also a full-featured statistics package NumPy, which is especially popular among data scientists. I have a forthcoming paper on the same topic (asylum policy) as the EUP article mentioned above.Since version 3.x Python includes a light-weight statistics module in a default distribution, this module provides a lot of useful functions for statistical computations. P.P.S On the internet, there is yet more confusion: for example, this document (which pops high in the Google results) has yet a different formula, shown in a slightly different form here as well.ĭisclaimer. To be clear, the weighted variance issue is not central to the argument of the article cited above but is significant as the authors discuss at length the methodology for estimating variability in data and introduce the so-called Coffey-Feingold-Broomberg measure of variability which the authors deem more appropriate for proportions. Here you can find the function Gavin Simpson has provided for calculating the weighted variance in R and try for yourself. When calculated properly, the weighted variance turns out to be 0.0008. You would guess that we can compute the weighted variance by analogy, and you would be wrong.įor example, the sample variance of ] equals 0.5(0.38-.0.40)^2+0.5(0.42-0.40)^2 =0.0004.Īs explained above, this is not generally correct unless the biased (population) rather than the unbiased (sample) weighted variance is meant. If we assign the weights 0.9 to the first observation and 0.1 to the second, the weighted mean is (0.9*0.4+0.1*0.8)/1, which equals to 0.44. For example the mean of 0.4 and 0.8 is 0.6. The weighted mean is just the mean but some data points contribute more than others. Things get tricky however when we want to calculate the weighted coefficient of variation.
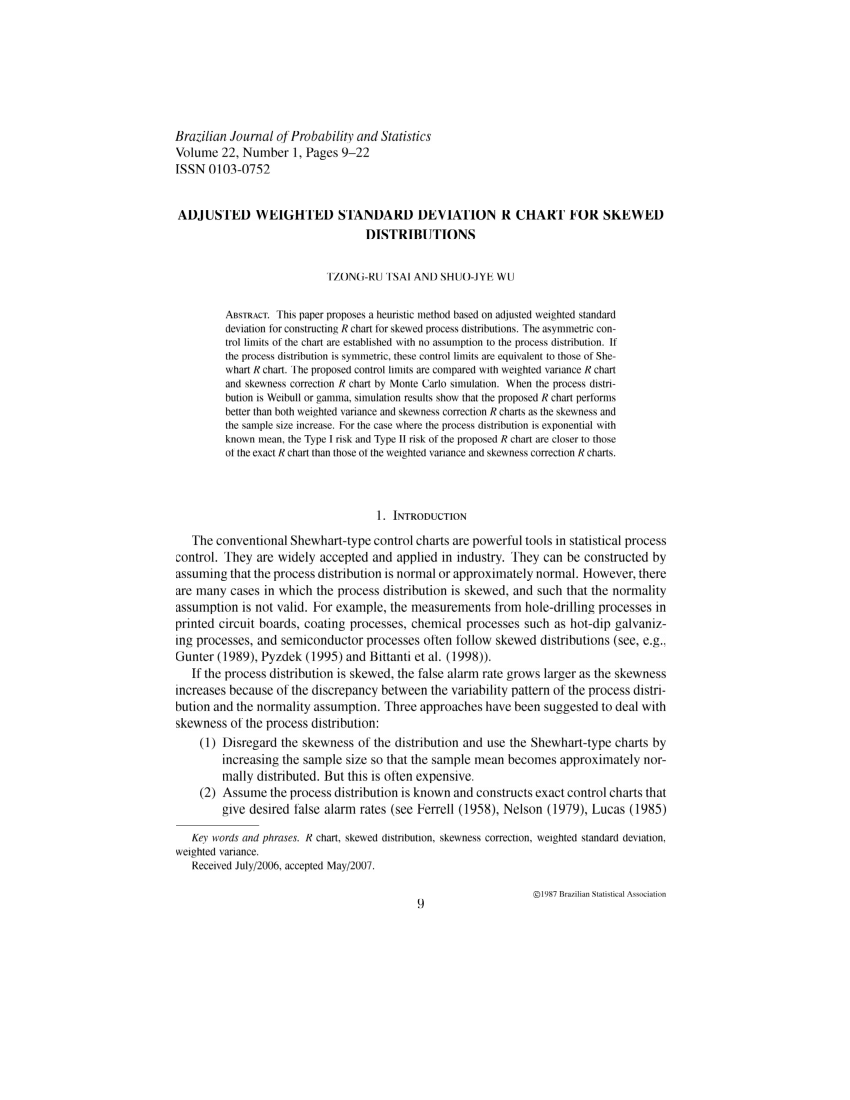
A common and easy fix is to use the coefficient of variation instead, which is simply the standard deviation divided by the mean. when you have counts of rare events) the mean equals the variance by definition. Clearly, comparing the variability of two Poisson distributions using the variance or the standard deviation would not work if the means of these populations differ. For example, when data is generated by a Poisson process (e.g. However, for some types of data, these measures are not entirely appropriate. The most often used measures of variability are the variance and the standard deviation (which is just the square root of the variance). Often we want to compare the variability of a variable in different contexts – say, the variability of unemployment in different countries over time, or the variability of height in two populations, etc.
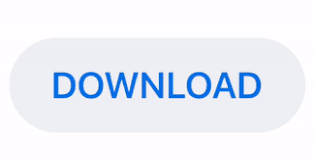